Most (93%) organizations say their fundraising teams understand how to use data for decision-making and are familiar with CRM systems, as reported in the 2025 CCS Philanthropy Pulse report. However, many still struggle with data accuracy and completeness. Over half (54%) of organizations identify incomplete or inaccurate data as a major obstacle to maximizing donor information. Similarly, 55% find it difficult to decide which analyses to run or lack the necessary training to do so. In this article, we explore nonprofit data management through actionable steps to improve your data quality by focusing on consistency, accuracy, and implementation. You’ll explore real-life examples of common data hygiene challenges, giving you practical insights to help bring your data to its healthiest, most reliable state.
Why Nonprofit Data Management Matters
For nonprofits, data is one of the most valuable assets. Strong data health—defined by accuracy, completeness, and consistency—ensures that information is a reliable driver of decision-making and operational efficiency. Well-maintained data saves time, reduces errors, and strengthens donor relationships. Clean and well-communicated fundraising data can also inspire meaningful action towards your mission.
Conversely, poor data health leads to inefficiencies, weakens engagement, and increases the risk of costly mistakes. By improving data quality, nonprofits can streamline operations, eliminate redundancies, and enable their teams to focus on what truly matters—their mission.
Questions to Ask About Your Data
- Is your database a reliable resource or a constant challenge?
- Are donor relationships effectively tracked through moves management?
- Do you have clear and consistent constituent codes, and are they applied uniformly?
- Is your biographical data, including prefixes, suffixes, and addresses entered in a standard format, such as consistently using “Apt.” for apartment?
- Are donor records accurate, complete, and regularly updated?
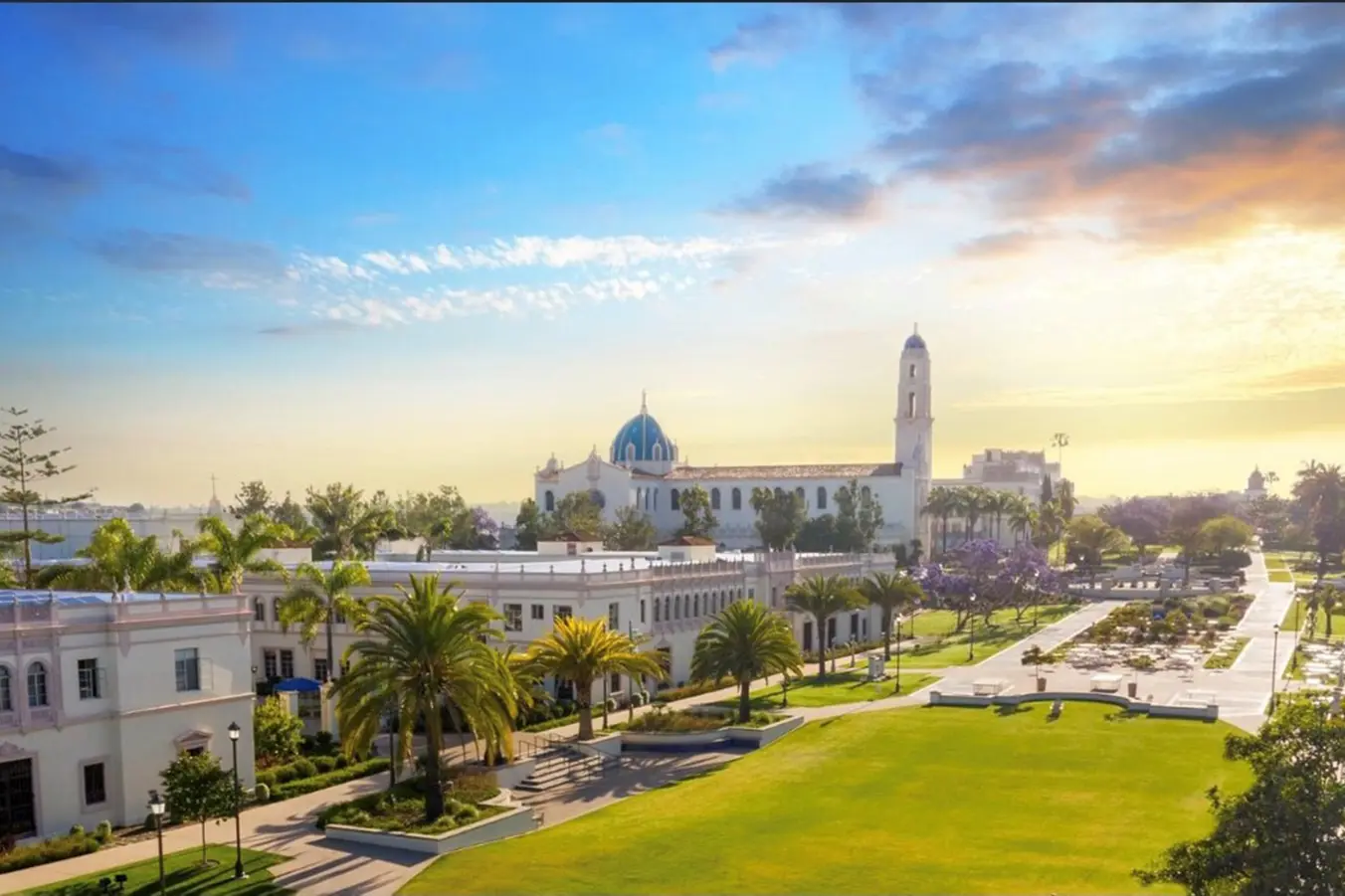
Read the university of san diego’s data analytics story
CCS’s Data Analytics team identified untapped philanthropic potential that made our ambitious campaign goal possible and provided the necessary insights to help us deploy campaign budget resources for maximum impact. This enabled a data driven decision-making process resulting in an actionable roadmap to campaign success and inspiring confidence and consensus among our staff, leadership, and board of trustees.
Richard P. Virgin, Ph.D., Vice President for University Advancement, University of San Diego
Common Challenges Observed in Nonprofit Data Management
We often hear nonprofits share that their data feels chaotic or inconsistent. Data in CRMs can be messy for many reasons: lack of clear standards, incomplete fields, or outdated information. Here are a few common issues:
- Abbreviations and Acronyms: Different users may enter “Street” as “St.,” “St,” or “Str.”
- Name Variations: “John Smith” entered as “John M. Smith” or “Smith, John.”
- Address Formats: State names entered as “CA” or “California.”
- Duplicate Records: Same donors being entered multiple times.
- Invalid Records: Rather than marking a record as inaccurate, the terms “Delete,” “Deceased,” or “Invalid Address” are added in front of the existing address.
- Unlinked Household Records: Donors from the same household with incomplete spouse fields or no clear primary contact/head of household indicator.
- Notes: Free form notes often lack structure, making it difficult to track key details.
Addressing these challenges requires clear policies, constant training, and regular audits to catch errors before they become problems.
What Characterizes Healthy Nonprofit Data Management?
Healthy data is a reliable foundation for decision-making, ensuring efficiency and stronger donor relationships.
Healthy Data Is:
- Accurate: Error-free and precise
- Complete: All key fields are filled in
- Uniform: Data is entered regularly and consistently in standardized formats
- Actionable: Data provides clear insights for decision-making
Notice how those characteristics are present in the below example of a healthy dataset (click on the three ellipses and then the desktop symbol to expand):
Steps to Get There:
- Conduct regular data audits to identify gaps or discrepancies
- Host ongoing team training on standardized processes
- Establish data cleaning routines to remove duplicates and correct errors
- Codify and update clear data management policies, such as a defined data dictionary, to ensure uniformity
Your development or advancement office may find it useful to implement a project management or Gantt chart, like the one below, to ensure clear coordination among key stakeholders. This includes the data manager, director of development, CRM administrator, development coordinator, development manager, executive director, and any external data consultants.
Month | Task | Description | Key Milestones |
---|---|---|---|
1 | Initiate Data Audit | Assess current data health, identify gaps, and prioritize fixes. Establish a baseline of current data status for quantifying improvement. | Data audit plan finalized |
2 | Review & Standardize Data Entry Practices | Create/update a data dictionary and standardize how data is entered and cleaned. | Data entry guidelines established |
3 | Train Staff on Data Processes | Provide training on data entry, CRM use, and standard operating procedures. | Training sessions completed |
4 | Initialize Data Cleaning | Remove duplicates, correct errors, and fill in missing key fields. | 50% of data fields cleaned |
5 | Implement Ongoing Quality Checks | Set up periodic reviews to ensure data accuracy and consistency. | First quality check completed |
6 | Monitor & Adjust Processes | Analyze progress, refine processes, and ensure adherence to best practices. | Data accuracy rate improved |
7+ | Establish Long-Term Maintenance Plan | Create a routine for audits, training, and continuous improvement. | Healthy data benchmarks met; data review calendar solidified |
Immediate Nonprofit Data Management Steps You Can Take
No matter where you are in your data health journey, there are actionable steps to strengthen and refine your approach.
Here are three common stages and the strategies to move forward:
- Stage One | The Aspirant: Beginning to address data health
- Clean up duplicates and standardize key fields
- Establish a simple, clear policy for uniform data entry
- Stage Two | The Architect: Being aware of data issues but struggling with consistency
- Implement regular audits and train staff on standardized processes
- Use dropdowns for entry of commonly repeated fields (e.g., states, prefixes) to reduce errors
- Stage Three | The Artificer: Looking to refine already-strong data practices
- Automate processes like deduplication and data validation
- Enhance donor tracking with more advanced moves management and constituent coding
By identifying your current stage and taking targeted steps, you can build a data system that supports efficiency, accuracy, and stronger donor relationships.

What are your data challenges?
We can make your job easier.
More Insights
Elevate Your Major Gifts Fundraising Training with These Three Tactics
Strengthen major gifts fundraising training at your organization through capacity-building, sustainable learning practices.
AI + Fundraising Webinar
Explore the transformational potential of artificial intelligence in modern fundraising practices in this video featuring leading experts and practitioners in the field.